There is a lot of talk about carbon emissions these days. But where does the data come from? Do you know your carbon emissions number?
For almost everyone, the answer is “no.”
Getting emissions data is hard. Scope 2 Emissions, which is the energy used in our buildings (electricity, natural gas, home heating oil and so on), comes from data on utility bills. There are over 3500 electric and gas utilities in the US and they each have a small catalogue of rates and bill layouts. There could easily be 35,000 document variations.
Most markets have a long tail of small players, but the US utility bill landscape is almost entirely a long tail! Every month a stack of bills per document variant is issued. But, as anyone who has dealt with utility bills has experienced, various unexpected elements appear: credits, new charges, corrected billing, a quarterly tax and so on. So the effective long tail is quite a bit longer. And month after month, one must process just a few bills per variant. There is never a stockpile of documents in the 1000s that can be used to train a large AI system.
So no surprise, standard AI, which is built on from training on thousands and thousands of similar documents, just doesn’t work. Standard AI also runs up significant computing, training and data acquisition costs. And they fail to automate more than 50% of the tail. (For details see the blog posts from A16z. To see what we mean by standard AI, see the description of AI used in the financial services sector here, in a report on AI issued by the London Stock Exchange.)
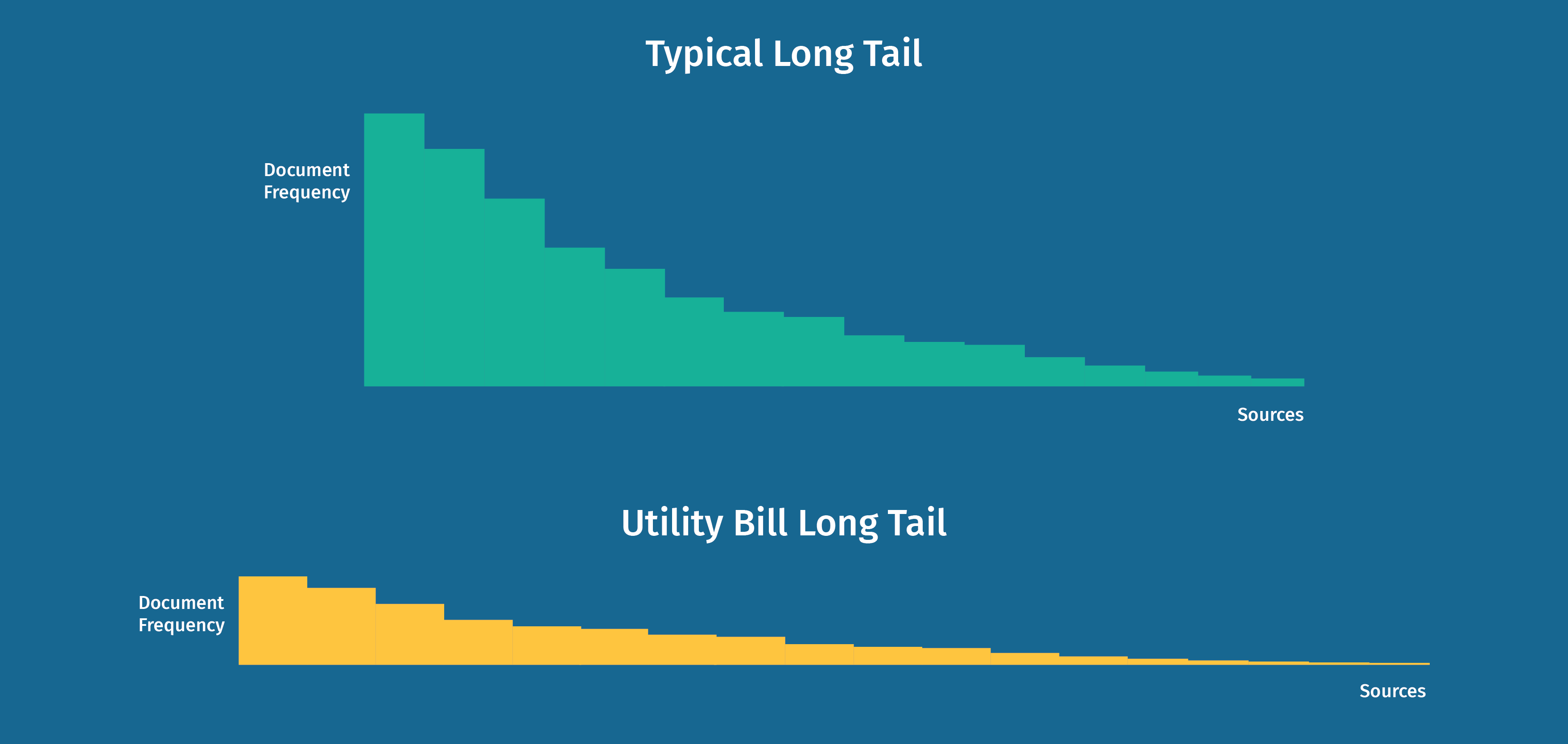
In the carbon emissions world, incomplete data has a huge cost. Consider, for example, the municipal electric utilities that serve about 20% of the US market. Definitely part of that long tail of utility bill variation, and with bill variants that have very low document counts. If a F5000 company happens to have a branch in that market, how do they get an automated, verifiable carbon emissions data flow? When standard AI fails to automate, the whole system chokes.
To solve this problem, GLYNT is built on a different kind of AI, known as “Few Shot” machine learning. It only takes three to seven sample documents to train GLYNT and deliver production-ready data at more than 98%+ accuracy. (See our annual accuracy report here.) We eat up long tails.
Now let’s turn to Scope 3 Emissions, which center on emissions from supply chains. These too have a long tail problem. 70% of supply chain transactions are triggered by PDF or paper invoices. The big players in the supply chain will be fully digitalized, but much of the ecosystem lives just fine on PDFs and paper. GLYNT’s advanced AI is the right solution here too.
The bottom line: Scope 2 and Scope 3 carbon emissions data is hard because key data is trapped in PDFs and paper documents, with enormous document variation. Standard AI is an incomplete solution. GLYNT was built for document flows that are a mile wide and an inch deep.
Get your Scope 2 Emissions Data from GLYNT.
Get Started with GLYNT